Could better connecting of data lead to a cure for Covid-19?
- 23 September 2020
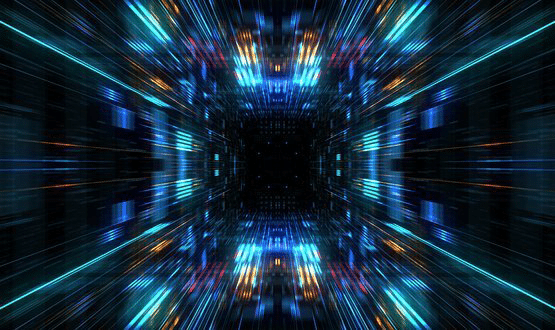
Large volumes of connected research data cannot be managed using classic analysis tools ā so scientists need to look for new approaches in combating major diseases, argues Dr Alexander Jarasch, head of data and knowledge Management at Munichās head-office of the German Centre for Diabetes Research.
The problem faced by anyone trying to study complex diseases like cancer, diabetes, heart disease and dementia is that the analysis methods we have relied upon have reached their limits. This is now a fact of life in scientific research, because the amount of data we collect is growing exponentially; just take the vast amount of data produced by novel research methods such as genomics as an example.
This difficulty is compounded by the interdisciplinary nature ofĀ life sciences research. To answer the really hard, but interesting biomedical questions about diabetes at my institution we have to connect data from many different studies, reports, surveys and research projects from different locations in Germany, including 500 researchers and 10 university hospitals.
This encompasses data from clinical trials and patient information, and our data covers various disciplines, from studies on a molecular level to pathway analyses and animal models. Clearly, itās no longer enough to answer a biological or medical question with ideas coming from one direction; we need to integrate and link more and more data. Doing that will be the next step in biomedicine and also in the healthcare sector, which is increasingly turning away from general blockbuster drugs and moving to individualised, precision medicine or treatment. For this effort to progress, it is necessary to network significantly more ā and above all look at as many aspects of the problem as possible.
Cross-disciplinary research
This is why a new approach to working with large, complex datasets, graph databases, could turn out to be crucial in helping in the prevention, discovery of new subtypes, early diagnosis and treatment of major illnesses. Our data workhorse, the relational database, still has an important role, but we need a technology to bring data silos together and uncover connections, as it presents a means to be able to jump from one data point to another.
Diabetes is a metabolic disease, so itās not sufficient for researchers to only look through metabolic data; we also have to take into account data from disciplines such as genomics or proteomics. In the human body, everything is connected in metabolic pathways; a gene encodes a protein that is active in a metabolic pathway and metabolises a metabolite, which in turn is able to regulate another gene. In a way, our metabolism is a network of thousands of components that are connected with each other, which is a graph data model. Thatās why itās so important to be able to uncover these connections and to create a new layer of analysis on top of this data, using graph technology.
We are using the same graph software, from Neo4j, to build a new Knowledge Graph to help fight Covid-19. The initiative connects data from a range of well-established public sources and links them in a searchable database, and itās helping researchers and scientists find their way through the 51,000-plus publications on the disease and related disease areas such as SARS, plus over 32,000 relevant patents. It allows them to create new hypotheses by querying not only literature information but also data on a gene or protein, clinical trial, drug and drug targets.Ā This is a critical capability in the absence of long term clinical trials and minimal peer reviewed research as we face the current pandemic.
An early breakthrough has been around ACE2
While we know a lot of data about genes, proteins and other entities, researchers are seldom aware of related research outside their field ā and no one can read that many papers and assimilate all that information, especially if we want to create effective Covid regimes and get to a vaccine as quickly as possible. Itās also a challenge to find key information that resides in different databases, because usually you have to carry out searches on the patent database, the publication database and the gene database, and then make the connections. Usually, researchers create Excel sheets, a list of identifiers and then they go to the database and then type in these identifiers, to get further information, but this yields limited results because of the lack of connections.Ā It is very manual work, error-prone, extremely inefficient and slow, also it misses non-obvious or indirect connections.
In contrast, the COVIDgraph.org is a graph database that allows us to structure this data and to connect it to the fundamental things from biology (e.g. genes, the proteins and their functions).
We have also just added a clinical trials database, to understand what kind of Covid-19 clinical trials are out there.Ā The data set specifies typical inclusion criteria, such as people under a certain age, or a specific risk group, like diabetic patients. This is valuable information that is usually scattered across different databases, and now we can bring it together and link it with everything else.
An early breakthrough has been around ACE2, the host cell receptor that mediates infection by SARS-CoV-2 (the coronavirus responsible for Covid-19). One might assume that the receptor ACE2 is just active in lung tissue, because one of the most vulnerable groups for the virus is patients with lung disease, but it turns out that of 55 human tissues in our database, the receptor is active in 53 of them. This means the ACE2 receptor can attack almost every tissue of your body, so now we know that any potential vaccine will need to be able to fight the virus in all of these different tissue areas.
It is our hope that surfacing details like this via our use of data will prove useful in the race to find a COVID-19 vaccine, as well as take us to the next level in precision medicine, prevention and treatment of diabetes.
1 Comments
DesAcc totally agrees with Dr Jaraschās view, we’ve been unlocking complex health data for over 27+ years and new approaches are needed to working with large, complex datasets. Whilst the relational database is useful, we need data ingest mechanisms that donāt presume structure of the data in order to ingest and query it.
Moving from the data warehouse Extract-Transform-Load model to an Extract-Load-Transform model is essential for being able to quickly make new correlations between seemingly disparate data items.
Comments are closed.